OMeR is an integrative e-infrastructure based on the 3 key components. This integration is rely on a modular strategy and an effort toward standardization in order to guaranty interoperability. Particular care will be taken to ensure OMeR interoperability with key resources like MetaboLights and virtual research environments (e.g. framework of the European project PhenoMeNal).
OMeR components are internationally acknowledged and involved in European infrastructures (ELIXIR, PhenoMeNal...). Moreover, they are key elements in collaborative project with national SMEs (e.g. MedDay Pharmaceuticals) and international research institutes (CEA, EBI ...) and to foster new academic and private partnerships.
Service offered by OMeR relies on three e-Resources strongly supported by INRA and hosted in the instituted data centres:
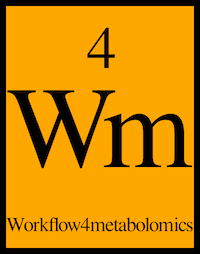
Workflow4Metabolomics, http://workflow4metabolomics.org
- First worldwide implementation of a metabolomics data analysis workflow system. W4M is currently a leading platform in Europe (Bioinformatics, 2014; IJBC, 2017) and is developed in collaboration with the CEA, CNRS, Sorbonne Université and Laberca.
- W4M is a key component in French infrastructures MetaboHub and IFB. Key resource in the H2020 European project "PhenoMeNal".
- Leading laboratory: INRA Ingenum - PI: Christophe Caron (IFB, MIA)
- Success indicators: 70 citations, >1,300 users, 90,000 jobs/year, 11 To available.
- Technology readiness level: 5-6
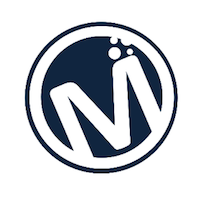
MetExplore, www.metexplore.fr
- Internationally pioneering project of a web server dedicated to the analysis of omics data in the context of metabolic networks, launched in 2009 (NAR, 2010; Bioinformatics 2017; NAR 2018).
- Key component of MetaboHUB and EU project PhenoMeNal.
- Leading laboratory: Toxalim - PI : Fabien Jourdan (MTH, ALIMH).
- Success indicators: 112 citations, > 500 users, >300 trained users.
- Technology readiness level: 5-6
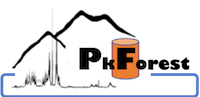
PeakForest, https://peakforest.org
- Central resource in MetaboHub allowing platforms to store and curate metabolic profiles PeakForest is also a centralized scientific knowledge warehouse in MetaboHub.
- Leading laboratory: UNH - PI: Franck Giacomoni (MTH, ALIMH/MIA)
- Success indicators: 6,000 metabolic profiles (MS and RMN) and 2,500 standard spectra, > 20 national curators.
- Technology readiness level: 3-4
Concerning technological aspects, OMeR is developing modular approaches, based on web components (e.g. MetExploreViz, Bioinformatics 2017) and microservices for phenotyping data portals (eg Food Metabolome Knowledge databases or Metabolic profiles of Biological matrices database). OMeR has a strong and broad expertise in the development of systems integrating SQL technologies, noSQL and built upon R frameworks, python, java and JavaScript. Several prototypes are under development like the work initiated with an INRA funding (15k€) for a « Virtual environment for global metabolism study ».
For methodological aspects, OMeR implements strategies for data life cycle management (storage, secured environment...) related to data production platforms (MetaboHub and beyond) and other partners. These strategies are currently integrated in the quality policy of CNOC INRA labeled metabolomics platforms. OMeR interacts with European partners (H2020 PhenoMeNal, JPI FOODBALL, ...) in the developments of SOP to create centralized data curation portals. OMeR also develops and implements original algorithms to analyze metabolic networks which constitute the biological context of metabolomics data. Future directions include text mining and semantic approaches to enrich knowledge associated with metabolomics data.